Precision Medicine Is the Future Of Healthcare
When it comes to applied hard sciences, a source of progress has been more precise measurements and tools. This is especially true in physics and chemistry, with analytical tools now able to observe individual atoms reactions to experiments, driving quick progress in clean energy, material sciences, nanotechnology, manufacturing, and computing (follow the links for articles on these topics).
However, one scientific field has proven to be a tougher nut to crack for precise measurements: biology. This is because living organisms are not “simple” materials made of a few elements but ultra-complex molecular machinery made of millions, if not billions, of different parts.
So, a true representation of a single human cell would be almost incomprehensibly complex, as illustrated by a computer-generated picture of a single human cell that went viral a few years ago.
Source: Newsweek
This has made a true understanding of biological and biochemical phenomena a persistent challenge.
Genomics has been an important first step, in explaining the template/instructions used by the cells to build their internal components. Another growing factor is the use of AI, as advanced neural networks are able to handle the massive amount of data better than the human mind.
Together, this will drive the transformation in healthcare, with the emergence of truly personalized precision medicine, tailored to each individual’s unique makeup of genes, metabolism, medical history, etc.
Currently, precision medicine is a $500B market, and includes monoclonal antibodies, (a $222B market in 2023), as well as most advanced cancer treatments like CAR-T therapies.
What is Multiomics?
The sheer complexity of living systems has led to the emergence of multiomics, a field merging together all the -omics sub-segments of biological sciences and touted as the next step in biotechnology:
- Genomics: the analysis of the DNA sequence in the cells’ nucleus.
- Transcriptomics: the analysis of mRNAcarrying the DNA’s instructions.
- Epigenomics: the modification of the genome without affecting the genetic sequence, or “epigenetics”.
- Proteomics: the analysis of proteins, including the modification of proteins with sugars (“post-translational”).
- Metabolomics: the analysis of chemical compounds and the metabolism.
- Microbiomics: the analysis of all the microbes living inside or on the body.
- Single-cell multiomics: the multiomics analysis on individual cells.
- Spatial biology: analyzing in 3D the location of specific mRNA, proteins, or cells.

Source: Ark Research
New fields are emerging, like, for example, Agrigenomics (genomics to improve agricultural yields), Ecological genomics (accurately evaluating the health of an ecosystem and its genetic diversity), or Synthetic Biology (creating new genes, traits, or entire organisms with a specific purpose).
Each of these fields has made tremendous advances thanks to new & improved analytical methods, in large part carried by revolutionary progress in nanotechnology, optics, semiconductor technology, and computing power.
Unfortunately, biological sciences and medicine have started to struggle to deal with all these new data, and even more to make sense of the complex interactions of every possible -omics field with each other.
In large part, this is due to literally trillions or maybe quadrillions of potential cross-interactions between individual genes, proteins, biomolecules, bacteria, etc.
And in theory, for truly personalized medicine, this data would be collected for every single individual, and put in connection to the records of their digital health data.
Declining Costs & A Flood of Data
The technological improvement in analytical tools has collapsed the costs of collecting new data. This is, for example, true for sequencing a full human genome, whose costs have been divided by more than a million in 30 years, or DNA synthesis is now 10,000x cheaper.

Source: Ark Research
It means that when it initially cost $450,000 to sequence only one genome in 2001, we now can sequence 1.4 billion genomes for the same price, or 17% of the world’s population.
As a result, genomics and other -omics data have been flooding biologists.
For example, the UK Biobank, the largest publicly-available genomics database, contains 27x times the data powering one of the largest LLM (Large Language Model), the AI Llama 405b built by Meta (FB +0%).
If every newborn in the world had its genome sequenced, a likely practice in the coming years, this would generate 10,000x the data used by Llama every year.

Source: Ark Research
Luckily, while the amount of data is multiplying by the thousands, so is the efficiency of digital analysis tools, especially AI, becoming 1,000x more powerful for the same cost.
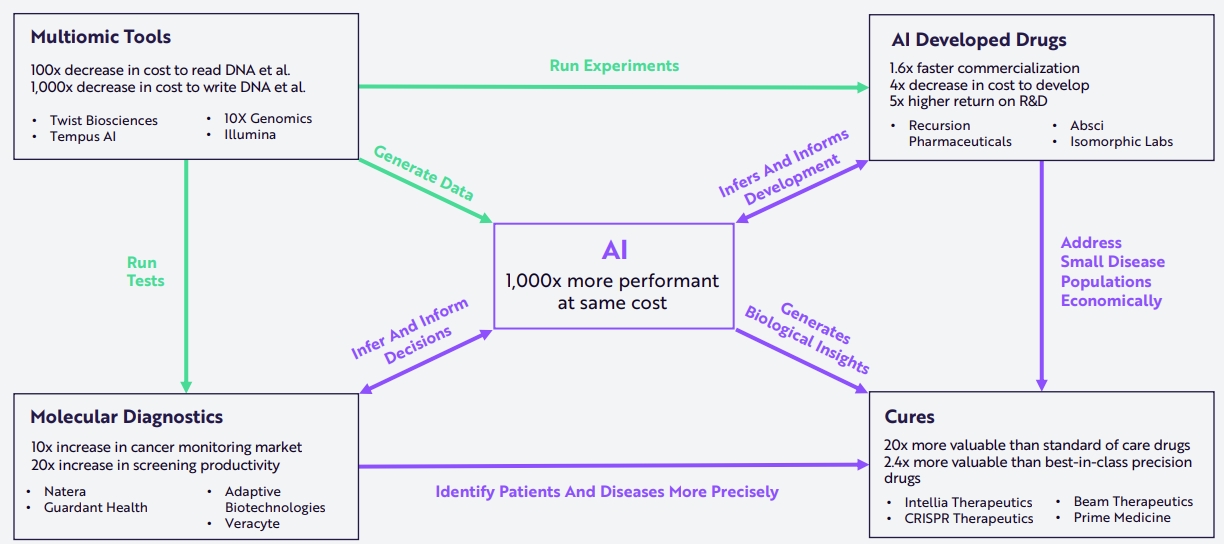
Source: Ark Research
Applications in Drug Discovery
Virtual Cells
Until recently, to know the effect of a potential new drug, or how a protein interacts with another, biologists needed to run the experiment manually, an expensive and time-consuming task.
This naturally slowed down new drug discovery and increased healthcare costs for innovative treatments. It could either be done in-vitro (in a lab) or in-vivo (in a living organism, usually an animal).
A new option has recently appeared, the in-silico approach, where one or several virtual cells are simulated in a computer. These virtual cells are then exposed to the potential new treatment and the simulation calculates how they would react.

Source: Ark Research
Improved Simulation
Besides full genome and transcriptome data, another tool is now making its way into in-silico simulation: protein folding simulators like Google’s AI AlphaFold (GOOGL -0.59%).
Many medicines based on proteins are dependent on the interactions between the drug and a receptor in the cells of the body, or the surface of targeted bacteria or cancer cells.
Correctly predicting in-silico the protein’s 3D configuration (folding) will radically improve the success rate and speed of drug development, driving costs down.
As AlphaFold improves by up to 500x since 2018, in-silico simulations will become a must-have technology for most biotech companies.

Source: Ark Research
Other companies are also working on similar technology to AlphaFold but for non-protein molecules, like Schrödinger , which we covered in “Top 5 AI & Digital Biotech Companies”.
Cancer Detection
The early detection of cancer, even when it is not visible in an MRI scanner, can often be a matter of life or death.
A new technique called liquid biopsy carries the promise of detecting early such invisible cancers. How it works is that it uses genome sequencing tools to detect DNA sequences typical of cancer in the patient’s blood, even if these sequences are ultra-rare.
Liquid biopsies are much less invasive than actual biopsies, requiring only a blood sample. They could also be used to look for multiple potential cancers at the same time.
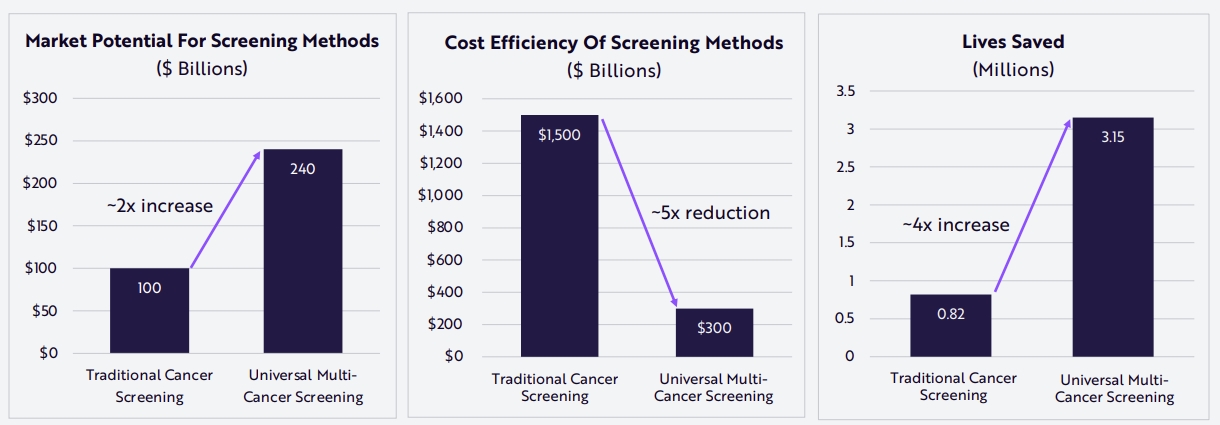
Source: Ark Research
In June 2024, Guardant Health (GH -2.25%) saw its “Shield” colorectal cancer screening test approved by the FDA. Grail (GRAL +0.99%), the recent spin-off from Illumina (ILMN -1.82%) is also a company developing such a test.
Besides liquid biopsy, Minimal residual disease (MRD) testing is also becoming increasingly common for checking the remission of cancer patients. MRDs can detect the recurrence of cancer up to 20 months earlier than traditional imaging.

Source: Ark Research
For both MRD and liquid biopsy, reimbursement by private and national health insurance systems will be key in speeding up their adoptions.
Automated Lab
As more and more data are needed, and the detection tools get cheaper, it is increasingly the labor from humans with PhD-level qualifications that become the limiting factors (both in costs and capacity) for generating more multiomics data.
This is true both for the manual work itself, like extracting samples, and for the design of the experiments.
An emerging alternative method is the self-driving lab (SDL). This combines robotics and automation to replace tedious and slower manual labor, creating high-throughput experimentation. It also adds LLMs to analyze data and design the next set of experiments.

Source: Ark Research
A leader in this new drug discovery method is Recursion Pharmaceuticals (RXRX +0.65%) (more on this company below).
Investment Insights
While there are many companies innovating at the intersection of AI and multiomics, a few stand out by either their importance or their ambitions.
Recursion Pharmaceuticals
Recursion Pharmaceuticals, Inc. (RXRX +0.65%)
Recursion is a company that, from its inception, was focused on using AI to accelerate new drug discovery. To do so, it combines dry lab (in-silico) and wet lab (biological samples) with:
- A library of 1.7 million small molecules.
- Cell cultures, CRISPR gene editing, soluble factors, live viruses, etc.
- An automated laboratory robotics workflow that allows for up to 2.2 million experiments each week.
- High-throughput microscopes and sequencing systems.
- Continuous video feeds from cameras, recording holistic measurements of animal behaviors.
- Advanced computational resources, which have generated >21 petabytes of proprietary high-dimensional data.
- ADMET (absorption, distribution, metabolism, excretion, and toxicology) data.
Recursion also owns one of the world’s fastest supercomputers to train their LLMs and AIs for drug discovery. The AI models were trained on a library of more than 2 billion images and inferred 6 trillion relationships between all possible combinations of genes and compounds.

Source: Ark Research
In August 2024, Recursion merged with Exscientia, a company focused on precision therapies and using its own “comprehensive robotic automation across the entire experimentation cycle”. Recursion also acquired in May 2023 the drug chemistry-focused preclinical startups, Cyclica and Valance, for a total of $87.5M.
With these newly acquired companies merging with Recursion’s core datasets, the company is now a fully integrated biotech company, handling everything from target identification, in-silico predictions, in-vivo validation, and clinical trials.

Source: Recursion
Recursion has 20+ molecules at various stages of development in its R&D pipeline, of which 7 are in stage 1/2 of clinical trials, mostly in oncology (cancer) and rare diseases.

Source: Recursion Pharmaceuticals
These programs include 10+ partnered programs with up to $20B in potential payment for R&D milestones, of which $450M have already been paid.

Source: Recursion Pharmaceuticals
Overall, the new Recursion, grown through acquisitions and an early move in leveraging AI for drug discovery, is shaping to become a key partner for large pharmaceutical companies looking to replenish their R&D pipeline.
Illumina
While the other -omics are important, almost all articulate one way or another around genomics, the core “instruction manual” of every living cell.
And by far, the largest producer of genome sequencing machines is Illumina. The company is focused on short genetic sequence reading, the one used for cancer detection. It currently has 22,000+ installed sequencers in 165 countries.
Around half of Illumina’s sequencing machines’ consumables are used in clinical applications, with the other half used in public and private research labs. In clinical applications, half of the demand comes from oncology.

Source: Illumina
As genomics and multiomics become the center of the drug discovery process, as well as cancer diagnostics, Illumina’s equipment is expected to be in high demand. The company expects the demand for NGS (Next Generation Sequencing) to grow by 18% CAGR for clinical applications and 6% CAGR for research, boosting the sector’s total addressable market (TAM) from $100B for clinical and to $25B for research by 2033.

Source: Illumina
Illumina had a complicated history with liquid biopsy company Grail (GRAL +0.99%), which was a spin-off from Illumina, later reacquired, and now forced back into a spin-off by competition authorities in the US and the EU.
With this trouble out of the way, Illumina might resume its long-term growth and stock price rise, especially as ultimately, Grail’s liquid biopsy tests will likely rely on Illumina sequencers.
Ginkgo Bioworks
Most of the multiomics-focused companies are present in the pharmaceutical/biotech space due to the potential profitability of blockbuster cancer treatments or curing rare diseases.
But this would be ignoring the incredible potential of biosystems for countless other applications, including chemical production, agriculture, materials, biofuels, etc.
This is exactly the focus of Gingko Bioworks, with an innovative model of building “organisms on demand” to answer specific industrial needs, making it a leader in the emerging field of synthetic biology (see “Top 5 Synthetic Biology Public Companies”).
This way, Gingko can offer any level of collaboration, from simply selling the tools to produce new organisms to contracting its solutions to full-fledged partnerships.
Among Gingko’s various research programs and partnerships can be mentioned:
Gingko’s expertise is demonstrated by the very diverse array of clients and partners the company has had over the last few years, from global industrial and agricultural companies to leading pharmaceuticals.

Source: Gingko Bioworks
The company’s research hardware is structured around the Reconfigurable Automation Carts (RACs), which form the modules that can integrated together into entire research labs for high-throughput biological data generation.
Gingko had to reform its business model in 2024, after a period of expansion and too many geographical locations. This has allowed the company to cut its operation expenditure (opex) from $515M per quarter to $375M, mostly by dividing overhead costs in half.
This should bring it closer to profitability, as well as further progress on research agreement with their corresponding payment milestones.
Challenges
Privacy
If the era of multiomics analyses and personalized medicine is coming soon, it is not without challenges. The very first one is the question of data privacy, an especially sensitive topic when the data are not just our digital life but our very own bodies.
It is clear that good security, access only to authorized personnel, and anonymization of data, as well as avoiding such data to be leveraged to deny medical care or insurance will be a must for people to embrace this technological revolution.
Concern about universally collected genetic data, especially if it is accessible by non-medical specialists (for example, members of the police or state apparatus) will also need to be addressed.
Regulations
Due to the sensitive nature of biological data, increased regulations are to be expected. This covers first the question of privacy, but also topics other topics like:
- The risks of monopoly emerging, with one or few companies progressively taking control over all our biological data, as the more data, the more efficient the AI-based analyses will be.
- Managing a balance between understanding risks for individuals or whole populations, and avoiding discriminations or unfair commercial practices.
- Fair treatment regarding personalized medicine no matter your wealth, but also managing the potential collective costs.
Accessibility
Because personalized medicine and multiomics data are extremely complex, they will be difficult to explain to non-scientists or doctors. Combined with the risks of unfairness and privacy concerns, it is likely that some resistance or even backlash will occur.
In the same way that the policy of mass vaccination with mRNA vaccine against Covid was highly politicized, such an outcome is not unlikely for multiomics and AI-driven medicine.
Similarly, such technology might at times be initially costly, and it will be important to avoid wealth inequality becoming a biological and health divide as well.
The Future Of AI-driven healthcare
The combination of a growing volume of medical data, powerful AI, automated biological labs, in-silico simulations of proteins, and even whole cells are creating entirely new fields of medicine and medical research.
It is also likely to be only the beginning, as more progress is piling up to speed up innovations even further:
Ultimately, in the not-so-distant future, most of medicine might be tailored to our individual genetic makeup, we might run a yearly foolproof cancer checkup from a blood test, and be more healthy and energetic thanks to the perfect balance of our metabolism, microbiome, and genome.
From an investment point, it is likely that the main winners in the AI-biotech race will be companies with the ability to create large biological datasets, more than having a unique proprietary algorithm or computation power, as AI technology is moving very fast beyond ultra-large datacenters providing a durable advantage, and more toward a decentralized open-source model.